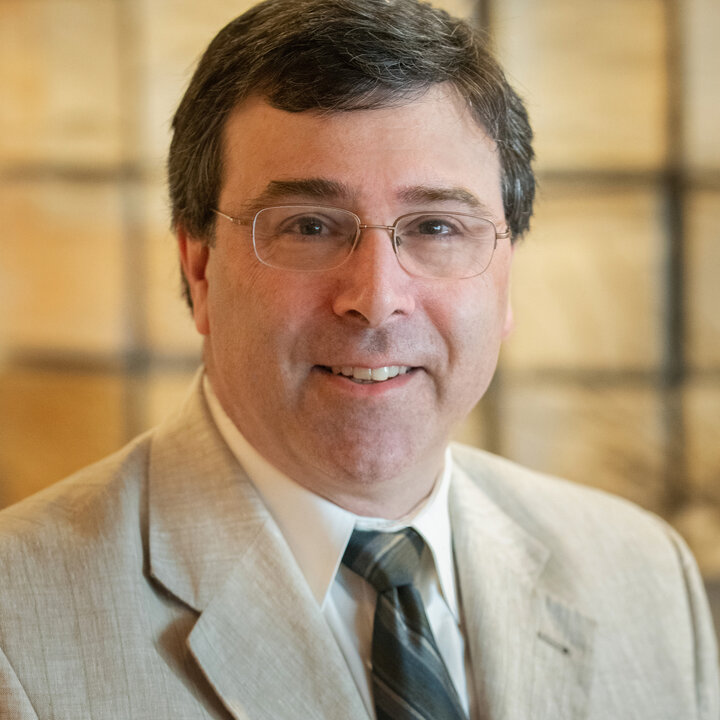
Bertrand Clarke
Professor Statistics University of Nebraska-Lincoln
Contact
- Address
-
HARH 354B
- Phone
-
-
Areas of Expertise:
Data mining and machine learning, prediction, statistical techniques for complex or high-dimensional data, model bias and uncertainty.
Research Areas of Interest:
My main interest these days is in prediction. This is broader than it sounds because prediction brings in questions about model uncertainty (Which model, if any, is true?) model mis-specification (If no model is true, what’s the least bad one?), model complexity (When is more complex modeling better than a simple approach?) and the other sources of variability and bias that have to be small enough for a prediction is useful. Obviously, different model classes can be used to generate predictors but there are also predictors that are not based on any model class. This is the case, for instance, with many machine learning methods such as bagging, boosting, kernel methods, and ensemble methods more generally. In these cases, it is reasonable to ask what the predictor means, i.e., what does a good predictor say about the properties of the phenomenon being predicted? Complex and high dimensional data are the natural places to use predictive techniques since model identification is so hard – even if one believes a model exists (often a dubious assumption). So, I tend to be interested in genomic or other types of complex data where useful formal theory is rare but statistical principles (variance-bias, robustness, complexity minimization, etc.) still provide helpful guidance. Analyzing complex data, or better, developing and understanding good predictors for complex data, often includes clustering, dimension reduction, complexity concepts, ensemble methods – and much else. Indeed, the predictive approach can be regarded as providing an overall conceptualization of the statistical problem in much the same way as Bayes, frequentist, survey sampling, or decision theory does.
Publications:
Statistics
Data Mining and Machine Learning
Biomedical
Predictive
Dustin, D, Clarke, B. (202?). A Conservation Law for Posterior Predictive Variance. In preparation.
Research interests:
My main interest these days is in prediction. This is broader than it sounds because prediction brings in questions about model uncertainty (Which model, if any, is true?) model mis-specification (If no model is true, what’s the least bad one?), model complexity (When is more complex modeling better than a simple approach?) and the other sources of variability and bias that have to be small enough for a prediction is useful. Obviously, different model classes can be used to generate predictors but there are also predictors that are not based on any model class. This is the case, for instance, with many machine learning methods such as bagging, boosting, kernel methods, and ensemble methods more generally. In these cases, it is reasonable to ask what the predictor means, i.e., what does a good predictor say about the properties of the phenomenon being predicted? Complex and high dimensional data are the natural places to use predictive techniques since model identification is so hard – even if one believes a model exists (often a dubious assumption). So, I tend to be interested in genomic or other types of complex data where useful formal theory is rare but statistical principles (variance-bias, robustness, complexity minimization, etc.) still provide helpful guidance. Analyzing complex data, or better, developing and understanding good predictors for complex data, often includes clustering, dimension reduction, complexity concepts, ensemble methods – and much else. Indeed, the predictive approach can be regarded as providing an overall conceptualization of the statistical problem in much the same way as Bayes, frequentist, survey sampling, or decision theory does.
Biosketch:
Bertrand Clarke earned his PhD in Statistics at the University of Illinois-Champaign-Urbana in 1989.His thesis work was given the Browder J. Thompson award for authors under age 30 of papers in IEEE journals. He spent three years as an Assistant Professor at Purdue University before moving to the University of British Columbia where he worked from 1992-2008. His early research focused on asymptotics, prior selection in Bayesian statistics, and mathematical modeling of biological systems. His first sabbatical was at University College London and his second sabbatical was at Duke University where he was a visiting scholar in the `Large P Small N’ program at SAMSI. In addition, in 2008 he spent three months at the Newton Institute at Cambridge University. He moved to the University of Miami in 2008 and worked for five years at the medical school where he started their MS and PhD programs in biostatistics before coming to Chair the Department of Statistics at the University of Nebraska-Lincoln. His current foci of research are predictive statistics and statistical methodology in genomic data. He has been an associate editor for four different journals, served three years on the Savage Award Committee (best thesis prize in Bayesian statistics), has published numerous papers over several fields, and was made a Fellow of the ASA in 2014. He has also authored one PhD level textbook on data mining and machine learning for Springer, with a complete solutions manual (available to instructors on request).
Education
Bertrand Clarke earned his PhD in Statistics at the University of Illinois-Champaign-Urbana in 1989.His thesis work was given the Browder J. Thompson award for authors under age 30 of papers in IEEE journals. He spent three years as an Assistant Professor at Purdue University before moving to the University of British Columbia where he worked from 1992-2008. His early research focused on asymptotics, prior selection in Bayesian statistics, and mathematical modeling of biological systems. His first sabbatical was at University College London and his second sabbatical was at Duke University where he was a visiting scholar in the `Large P Small N’ program at SAMSI. In addition, in 2008 he spent three months at the Newton Institute at Cambridge University. He moved to the University of Miami in 2008 and worked for five years at the medical school where he started their MS and PhD programs in biostatistics before coming to Chair the Department of Statistics at the University of Nebraska-Lincoln. His current foci of research are predictive statistics and statistical methodology in genomic data. He has been an associate editor for four different journals, served three years on the Savage Award Committee (best thesis prize in Bayesian statistics), has published numerous papers over several fields, and was made a Fellow of the ASA in 2014. He has also authored one PhD level textbook on data mining and machine learning for Springer, with a complete solutions manual (available to instructors on request).